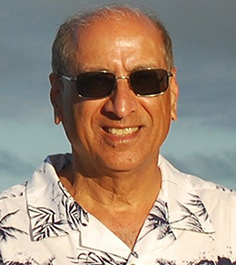 |
Workshop Introduction and Objectives
Jay Lala, Raytheon Technologies
Abstract: The IFIP Working Group 10.4 (Dependable Computing and Fault Tolerance) has recently undertaken a project on Intelligent Vehicle Dependability and Security (IVDS). The project vision, mission and goals are described on the home page of this workshop. The goal of this first IVDS workshop is to debate and provide arguments on all sides of the following hypothesis:
Level 3 autonomous vehicles cannot be made acceptably safe with current technology and practices.
The expected outcome of the workshop is a set of specific actions, both short term and long term, to achieve the IVDS project's vision, mission and goals. The context for the IVDS project and the workshop is the promise of safe and secure SAE Level 5 vehicles. In an ideal end-state, these vehicles will obviate the need for human drivers and even personal autos. Benefits range from no more human carnage on the roads to reclaimed home and business garages and parking lots, leading to green and clean cities. But none of these benefits will be realized unless the vehicles are safe. Current thinking of industry leaders and some regulatory agencies is to make these vehicles at least as safe as human drivers. Unfortunately, this is a very low bar, and will lead to about 37,000 deaths (US) per year, due to machine failures. We will blow this once-in-a-century opportunity to make land transportation totally safe and reap all the potential benefits. Industry is also moving progressively from L0 to L5 autonomy which is counter-intuitively less safe than skipping mixed mode L3 and L4 and jumping straight to totally driverless L5. The introduction will set this context for the workshop presentations, panel and discussions.
Bio: Dr. Jaynarayan Lala is a Senior Principal Engineering Fellow at Raytheon Technologies. He is the Cyber Technology Director for the Raytheon Missiles and Defense (RMD) business unit where he has led advanced cyber technology development for the past 17 years. He is now also serving as the RMD Product Cybersecurity Officer. Jay has 45 years of experience that spans industry, government and research & development labs. At DARPA, he initiated ground-breaking research in intrusion-tolerant and self-healing systems. He was honored with the Secretary of Defense Medal for Exceptional Public Service for his work at DARPA. Prior to DARPA, for spent nearly a quarter century at Draper Lab in Cambridge, MA, where he architected fault-tolerant computers for many mission- and safety-critical platforms, including Seawolf Submarine, aircraft engine controllers and spacecraft. Jay has authored over 50 peer-reviewed publications, books and book chapters and has five patents. He (along with two Draper colleagues) received the IFIP Jean Claude Laprie award in 2015 for his work on the Fault Tolerant Multi-Processor (FTMP). Jay is a Life Fellow of IEEE and an Associate Fellow of AIAA. Jay received Doctor of Science and Master of Science degrees from MIT in Aero & Astro and a Bachelor of Technology (Honors) from Indian Institute of Technology, Bombay.
|
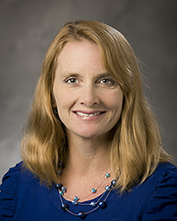 |
Tesla Model 3 Reliability in Driver Alerting
Missy Cummings, Duke University
Abstract: Automated driving assistance systems provide many comforts and conveniences, but they also can lead to complacency and distraction, which increases the need for effective driver alerting and monitoring. To this end, the results of a series of Tesla Model 3 tests focused on Autopilot and driver alerting will be presented, which show high variability between different Model 3s as well as within a single vehicle. Such high variability is suggestive of perception system problems and a need for industry-mediated standards.
Bio: Professor Mary (Missy) Cummings received her B.S. in Mathematics from the US Naval Academy in 1988, her M.S. in Space Systems Engineering from the Naval Postgraduate School in 1994, and her Ph.D. in Systems Engineering from the University of Virginia in 2004. A naval pilot from 1988-1999, she was one of the U.S. Navy's first female fighter pilots. She is currently a Professor in the Duke University Electrical and Computer Engineering Department, and the Director of the Humans and Autonomy Laboratory. She is an AIAA Fellow, a member of the Defense Innovation Board and a member of the Veoneer, Inc. board.
|
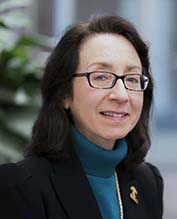 |
Approaches to Assessing and Communicating about AV Safety
Marjory Blumenthal, RAND
Abstract: Public trust in automated vehicles (AVs) can build on meaningful assessment and effective communication about AV safety. Each of these presents challenges and opportunities. My remarks will draw on a pair of projects conducted over the past three years and drawing heavily from semi-structured interviews with AV developers and other experts and stakeholders. Our research indicates that there are three principal approaches to assessing AV safety: measurement, processes, and thresholds. None stands alone–rather, they complement each other, speaking to gaps in what is known, and none is stable–they evolve over time, and so does the way they interact. There are important opportunities for industry to converge on how to present AV safety assessments in consistent and comparable ways. Public reactions to AVs are colored by how people perceive risk, which can be very subjective. Our most recent research included a novel survey of how the public responds to different sources of information about AV safety. It underscored the importance of messages that are data-driven and that come from objective sources.
Bio: Marjory S. Blumenthal, Senior Policy Researcher, joined the RAND Corporation as director of the experimental Science, Technology, and Policy program in spring 2016, with a broad remit that includes science and technology trends, societal impacts, and policy. Her work at RAND has addressed such topics as automated vehicles, smart cities, measuring the impact of research, innovation in China, and trends and applications of artificial intelligence, 5G, and other emerging technologies. As founding executive director of the Computer Science and Telecommunications Board (CSTB) at the US National Academies of Sciences, Engineering, and Medicine, she addressed the full range of information technologies and their impacts and is recognized for her work on the evolution of the Internet and cybersecurity. In 2003, she took a leadership position at Georgetown University, developing academic strategy, promoting innovation in teaching and learning, and fostering research, especially in the sciences. In 2013-2016, Marjory was executive director of the President's Council of Advisors on Science and Technology within the White House Office of Science and Technology Policy. Marjory has authored/edited numerous books and articles and serves on a variety of boards and advisory bodies. She did her undergraduate work at Brown University and her graduate work at Harvard University.
|
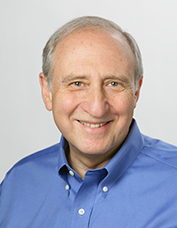 |
Designing for Increased Autonomy & Human Control
Ben Shneiderman, University of Maryland
Abstract: How can we understand failures and near misses from automobile data recorders? Who owns the data for retrospective forensic analysis? How to manage open reporting of failures? Could Self-Driving Car Control Centers improve dependability? The Human-Centered Artificial Intelligence (HCAI) model clarifies how to (1) design for high levels of computer automation and high levels of human control so as to increase human performance, (2) understand the situations in which full human control or full computer control are necessary, and (3) avoid the dangers of excessive human control or excessive computer control.
Bio: Ben Shneiderman is an Emeritus Distinguished University Professor in the Department of Computer Science, Founding Director (1983-2000) of the Human-Computer Interaction Laboratory (http://hcil.umd.edu), and a Member of the UM Institute for Advanced Computer Studies (UMIACS) at the University of Maryland. He is a Fellow of the AAAS, ACM, IEEE, and NAI, and a Member of the National Academy of Engineering, in recognition of his pioneering contributions to human-computer interaction and information visualization. His widely-used contributions include the clickable highlighted web-links, high-precision touchscreen keyboards for mobile devices, and tagging for photos. Shneiderman's information visualization innovations include dynamic query sliders for Spotfire, development of treemaps for viewing hierarchical data, novel network visualizations for NodeXL, and event sequence analysis for electronic health records. Ben is the lead author of Designing the User Interface: Strategies for Effective Human-Computer Interaction (6th ed., 2016). He co-authored Readings in Information Visualization: Using Vision to Think (1999) and Analyzing Social Media Networks with NodeXL (2nd edition, 2019). His book Leonardo's Laptop (MIT Press) won the IEEE book award for Distinguished Literary Contribution. The New ABCs of Research: Achieving Breakthrough Collaborations (Oxford, 2016) describes how research can produce higher impacts.
|
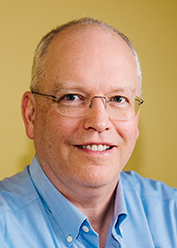 |
Automated Vehicle Safety Overview for 2021
Philip Koopman, CMU, Edge Case Research
Abstract: Various types of vehicle automation are being deployed on public roads, including vehicles that do not have continuous human driver supervision. This talk summarizes the state of safety in this industry, covering: types of vehicle deployments, what "safe" might mean for current deployments, challenges with human driver involvement, technology challenges, a snapshot of the standards landscape, and what it might take to deploy this technology at scale safely.
Bio: Prof. Philip Koopman, Ph.D., Co-founder and Chief Technology Officer of Edge Case Research, is an internationally recognized expert on Autonomous Vehicle (AV) safety who has worked in that area at Carnegie Mellon University for 25 years. He is also actively involved with AV safety policy, regulation, implementation, and standards. His pioneering research work includes software robustness testing and run time monitoring of autonomous systems to identify how they break and how to fix them. He has extensive experience in software safety and software quality across numerous transportation, industrial, and defense application domains including conventional automotive software and hardware systems. He served as the lead author of the ANSI/UL 4600 standard for autonomous system safety. Edge Case Research was founded by Michael Wagner and Philip Koopman, recognized world leaders in autonomous system safety as a trusted, independent third-party source to assess and develop safe autonomous systems. Established in 2014 with a renowned team of leaders in self-driving car projects with successes dating back to where it all began - Carnegie Mellon in the 1990s. Edge Case offers products and services built on best practices to reduce time to market and the cost of validation while still achieving a robust safety goal. https://www.ecr.ai
|
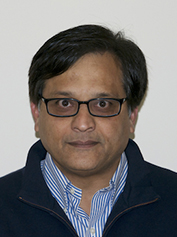 |
Diverse Redundancy and Testability: Key Drivers for Intelligent Vehicle Dependability
Nirmal Raj Saxena, NVIDIA
Abstract: Autonomous or self-driving cars initiative is creating a new center stage for resilient computing and design for testability. This is very apparent from reading the ISO26262 specification, which is about the functional safety for automotive equipment applicable throughout the lifecycle of all automotive electronic and electrical safety-related systems. By way of a brief review of the ISO26262, this panel talk pays tribute to all of the important and significant ideas that have come through the past 40+ years of research in fault-tolerant computing and design-for-testability. Among other components in a drive system, deep neural networks use the computational power of massively parallel processors. Autonomous driving demands extremely high resiliency and trillions of operations per second of computing performance to process sensor data with extreme accuracy. We show that design verification, testability and diverse redundancy help pave the path for autonomous vehicle dependability.
Bio: Nirmal R. Saxena is currently a distinguished engineer at NVIDIA and is responsible for high-performance and automotive resilient computing. From 2011 through 2015, Nirmal was with Inphi Corp. as CTO for Storage & Computing and with Samsung Electronics as Sr. Director working on fault-tolerant DRAM memory and storage array architectures. During 2006 - 2011, Nirmal held roles as a Principal Architect, Chief Server Hardware Architect & VP at NVIDIA. From 1991 through 2009, he was also with Stanford University's Center for Reliable Computing and EE Department as Associate Director and Consulting Professor, respectively, where he taught courses in Logic Design, Computer Architecture, Fault-Tolerant Computing, supervised six PhD students and was co-investigator with Professor Edward J. McCluskey on DARPA's ROAR (Reliability Obtained through Adaptive Reconfiguration) project. Nirmal was the Executive VP, CTO, and COO at Alliance Semiconductor, Santa Clara. Prior to Alliance, Nirmal was VP of Architecture at Chip Engines. Nirmal has served in senior technical and management positions at Tiara Networks, Silicon Graphics, HaL Computers, and Hewlett Packard. Nirmal received his BE ECE degree (1982) from Osmania University, India; MSEE degree (1984) from the University of Iowa; and Ph.D. EE degree (1991) from Stanford University. He is a Fellow of IEEE (2002) and was cited for his contributions to reliable computing.
|
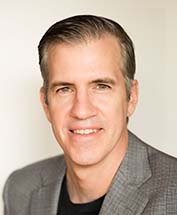 |
A Flexible, Verifiable, and Validateable Approach to Autonomous Vehicle Safety
Paul Perrone, Perrone Robotics
Abstract: The development of fully autonomous vehicles by organizations commands extraordinary multidisciplinary effort. Bringing responsive human level driving capabilities into ground vehicles to navigate complex environments without human intervention has yet to be deployed at scale in a production capacity. To address complexity, engineering efforts have wound higher level programming methodologies and artificial intelligence (AI) technologies into their solutions. However, traditional automotive verification and validation (V&V) techniques do not address nor do they scale for use of such approaches where "software stacks" bring an exponential increase to V&V cost and probabilistic AI technologies produce products with no deterministic V&V solution. This talk provides an overview of an approach for the V&V of autonomous vehicles for safety that squarely addresses such complexity and intractability. An independent safety monitoring process is presented which produces a verifiable and validateable approach to safety that is comprehensive, bounded in cost, and that is also flexible to address changing demands and scaling complexity. What's more, this verifiable and validateable approach to safety also may be leveraged for the cyber-security of autonomous vehicles as well.
Bio: Paul Perrone is founder & CEO of Perrone Robotics. He is the inventor of "MAX", the world's first general-purpose robotics operating system for autonomous vehicles along with novel claims in robotics and AV safety approaches (patented in 2006). He's been an early pioneer in the autonomous vehicle space leading Perrone Robotics for over 17 years in pioneering showcase achievements such as leading a team in the 2005/2007 DARPA Grand Challenges (autonomous vehicle races), early work with rocker Neil Young on vehicle automation, and creating rapid one-day drop-in autonomy solutions. He spearheaded the Company's Series A capital raise in 2016 (Intel Capital leading) and has continued to lead the company during its acquisition of flagship Fortune 500 customers including an automotive OEM, tier 1 automotive supplier, industrial equipment OEM, personal computer OEM, and automotive channel partner. He is currently leading the company's commercial deployment of autonomous shuttles for the transit of people and things via Perrone Robotics' "TONY" autonomous shuttle technology. He has 17+ years autonomous vehicle experience and 25+ years total hi-tech industry experience. His blend of experiences lies in the development of high-tech, business, and operations.
|
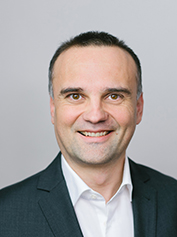 |
Coopetition as Enabler for Safe Self-Driving Cars and the Need for Scientific Foundations
Wilfried Steiner, TTTech
Abstract: Research and development of self-driving cars continues as a top priority in the automotive industry. However, meeting adequate dependability requirements remains a challenge. On the one hand the need for cutting-edge technology demands new forms of system design and dependability assurance techniques, on the other hand dependability requirements are not consolidated and agreed within the industry in the first place. We, therefore, believe that a much closer cooperation between competing industry stakeholders is necessary that goes beyond typical standardization activities and a well-balanced form of "coopetition" is required. Such a coopetition model is the goal of the "The Autonomous" initiative and in my talk, I will discuss the initiative's results and planned activities to achieve global reference solutions for safe self-driving cars.
Bio: Wilfried Steiner is the Director of the TTTech Labs which acts as center for strategic research as well as the center for IPR management within the TTTech Group. Wilfried Steiner holds a degree of Doctor of Technical Sciences and the Venia Docendi in Computer Science, both from the Vienna University of Technology, Austria. His research is focused on dependable cyber-physical systems, for example in the following domains: automotive, space, aerospace, as well as new energy and industrial automation. Wilfried Steiner designs algorithms and network protocols with real-time, dependability, and security requirements. Wilfried Steiner has authored and co-authored over eighty peer-reviewed scientific publications and is inventor and co-inventor of over thirty patent families. Wilfried Steiner has successfully contributed to multiple national and international publicly funded research projects. In particular, from 2009 to 2012 Wilfried Steiner has been awarded a Marie Curie International Outgoing Fellowship hosted by SRI International in Menlo Park, CA. Wilfried Steiner has also acted as editor for the SAE AS6802 standard (Time-Triggered Ethernet), has served multiple years as voting member in the IEEE 802.1 working group that standardizes time-sensitive networking (TSN), and is currently member in the ISO TC 22 that develops standards for safe autonomous road vehicles.
|
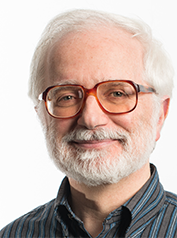 |
Trustworthy quantitative arguments for the safety of AVs: challenges and some modest proposals
Lorenzo Strigini, Citi, University of London
Abstract: Proving that accidents will be very rare is an essential part of arguing safety. Rigorous statistical and probabilistic reasoning is required. Autonomy poses various challenges, including system novelty, extreme requirements, AI-based implementations that undermine established verification methods. This talk will discuss possible quantitative requirements, the gaps between statistical evidence obtainable from testing before operation and safety levels to be demonstrated; and ways that other evidence can be rigorously combined with it to improve the confidence in vehicle safety. Proper statistical reasoning does not obviate lack of evidence, but can be made to exploit as well as possible what evidence is available, give confidence in the quantitative claims made, help steer verification activities and possibly indicate practical ways for supporting gradual adoption of autonomy.
Bio: Lorenzo Strigini has worked for approximately forty years in the dependable computing area. He is a professor and the director of the Centre for Software Reliability at City, University of London. He joined City in 1995, after ten years with the National Research Council of Italy. His career has included sabbatical visits at UCLA, Bell Communication Research, and SRI International. His work has mostly addressed fault tolerance against design faults, as well as against human error and against attacks, in various technical and socio-technical systems; and probabilistic assessment of system dependability attributes to provide insight, steer design and support acceptance decisions. He has published on theoretical advances as well as applied studies in application areas including safety systems for nuclear reactors, computer-aided medical decisions, the decision making about acceptance of safety-critical systems, autonomous vehicles.
|
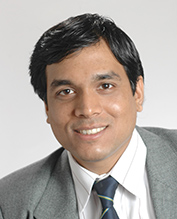 |
Verified Artificial Intelligence and Autonomy
Sanjit A. Seshia, University of California, Berkeley
Abstract: Verified artificial intelligence (AI) is the goal of designing AI-based systems that have strong, verified assurances of correctness with respect to mathematically-specified requirements. This goal is particularly important for autonomous and semi-autonomous systems. In this talk, I will consider Verified AI from a formal methods perspective and with a special focus on autonomy. I will describe the challenges for and recent progress towards attaining Verified AI, with examples from the domain of intelligent cyber-physical systems, with a particular focus on autonomous vehicles and aerospace systems.
Bio: Sanjit A. Seshia is a Professor in the Department of Electrical Engineering and Computer Sciences at the University of California, Berkeley. He received an M.S. and Ph.D. in Computer Science from Carnegie Mellon University, and a B.Tech. in Computer Science and Engineering from the Indian Institute of Technology, Bombay. His research interests are in formal methods for dependable and secure computing, with a current focus on the areas of cyber-physical systems, computer security, machine learning, and robotics. He has made pioneering contributions to the areas of satisfiability modulo theories (SMT), SMT-based verification, and inductive program synthesis. He is co-author of a widely-used textbook on embedded, cyber-physical systems and has led the development of technologies for cyber-physical systems education based on formal methods. His awards and honors include a Presidential Early Career Award for Scientists and Engineers (PECASE), an Alfred P. Sloan Research Fellowship, the Frederick Emmons Terman Award for contributions to electrical engineering and computer science education, the Donald O. Pederson Best Paper Award for the IEEE Transactions on CAD, and the IEEE Technical Committee on Cyber-Physical Systems (TCCPS) Mid-Career Award. He is a Fellow of the IEEE.
|
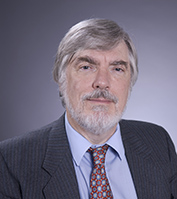 |
Model-Centered Assurance for Safe Autonomy
John Rushby, SRI International
(Joint work with Susmit Jha and N. Shankar)
Abstract: The functions of an autonomous system such as a self-driving car can generally be partitioned into those concerned with perception and those concerned with action. Perception builds and maintains a model of the world (e.g., the local road layout with nearby vehicles and pedestrians) that is used to plan and execute actions to accomplish a goal established by human supervisors (e.g., "take me to work"). Accordingly, assurance decomposes into two parts: a) ensuring that the actions are safe (and effective), given the model. and b) ensuring that the model is an accurate representation of the world as it evolves through time. Both perception and action may employ AI, including machine learning (ML), and these present challenges to assurance. However, it is usually feasible to monitor and guard actions with traditionally engineered and assured monitors, and thereby ensure safety, given the model. Thus, the model is the central focus for assurance. Traditionally, the model is derived bottom-up from sensors using AI and ML and this is known to be, and is bound to be, fault-prone. Instead, we propose an architecture that reverses this process and uses the model to predict sensor interpretation. Small prediction errors indicate the world is evolving as expected and the model is updated accordingly. Large prediction errors indicate surprise, which may be due to errors in sensing or interpretation, or to unexpected changes in the world (e.g., a pedestrian steps into the road). The former initiate error masking or recovery, while the latter requires revision to the model. Higher-level AI functions within the architecture assist in diagnosis and execution of these tasks. Although this two-level architecture where the lower level does "predictive processing" and the upper performs more reflective tasks, both focused on maintenance of a world model, is derived by engineering considerations, it also matches a widely accepted theory of human cognition.
Based on a SafeComp 2020 paper, which can be found here, including a recorded talk: http://www.csl.sri.com/users/rushby/abstracts/safecomp20
Bio: Dr. John Rushby is a Distinguished Senior Scientist and SRI Fellow with the Computer Science Laboratory of SRI International in Menlo Park California, where he performs research in assurance and formal methods for safe and dependable systems. He joined SRI in 1983 and served as director of its Computer Science Laboratory from 1986 to 1990 and as leader of its Formal Methods Program from 1990 to 2015. Prior to that, he held academic positions at the Universities of Manchester and Newcastle upon Tyne in England. He received BSc and PhD degrees in Computing Science from the University of Newcastle upon Tyne in 1971 and 1977, respectively. John Rushby received the IEEE Harlan D Mills Award in 2011 "for practical and fundamental contributions to Software & Hardware Reliability with seminal contributions to computer security, fault tolerance, and formal methods" and, together with Sam Owre and N. Shankar, the CAV Award in 2012 "for developing the PVS Verification which, due to its early emphasis on integrated decision procedures and user friendliness, significantly accelerated the application of proof assistants to real-world verification problems." His papers and presentations are available at: http://www.csl.sri.com/users/rushby/biblio.html
|